Dive into the transformative power of data observability! Join us to explore how it boosts data quality, empowers decision-making, and unlocks your data’s true potential.
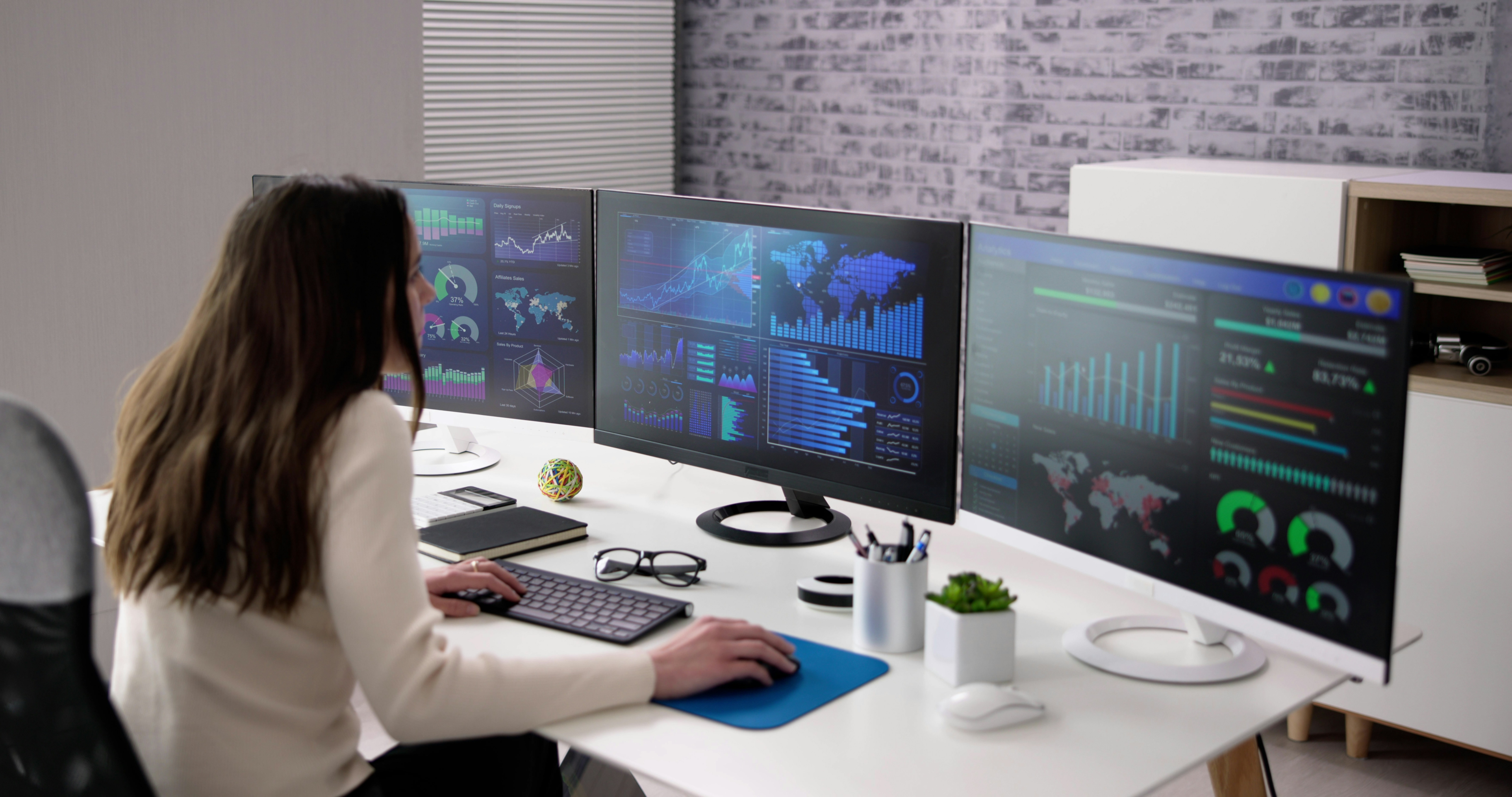
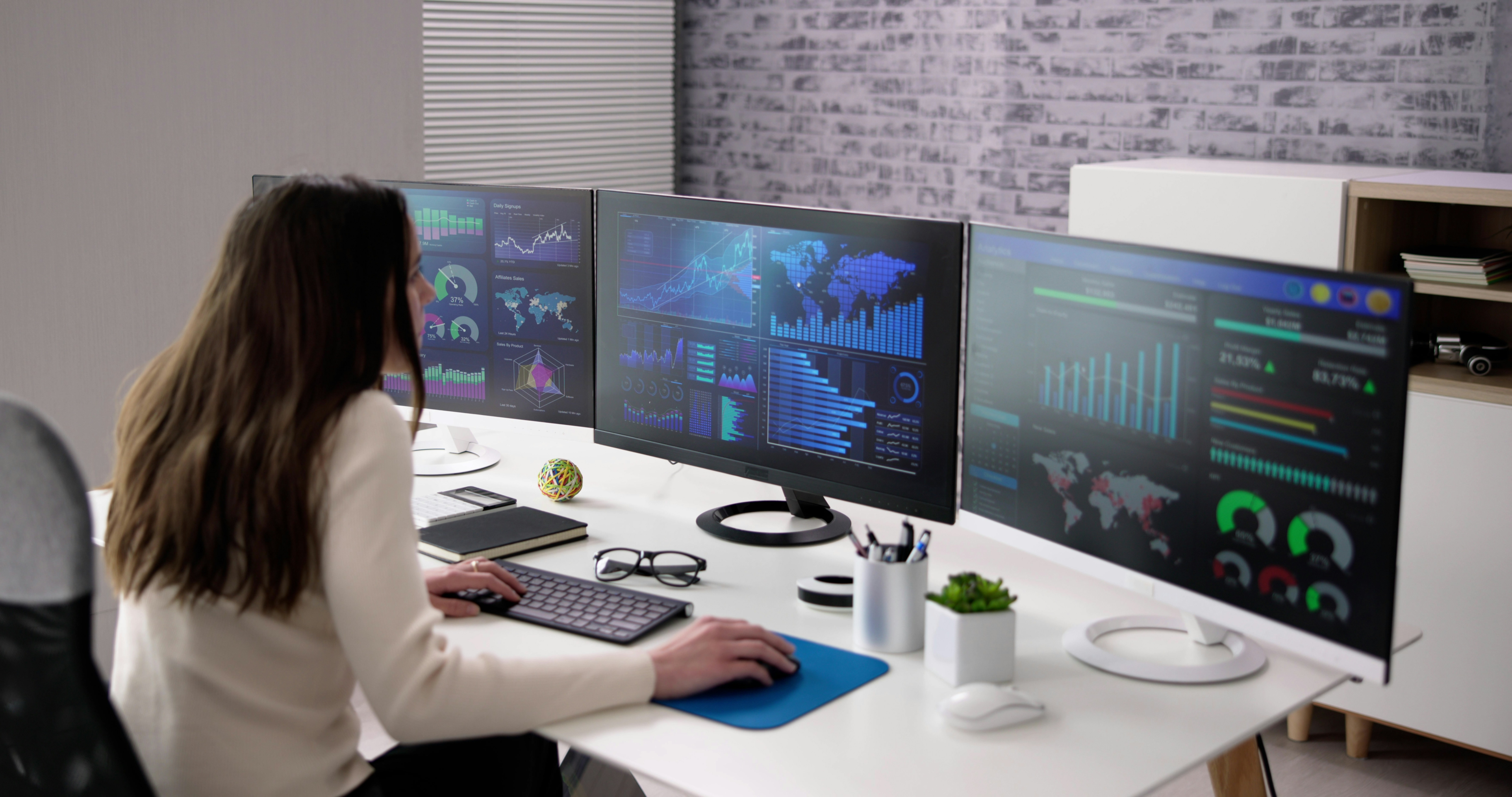
In our swiftly changing digital era, monitoring, analyzing, and understanding the data within our systems is more crucial than ever.
As we navigate through the complexities of software development and testing, observability emerges as a beacon of insight. It guides us toward higher data quality, enhanced performance, and ultimately, more reliable products.
Let’s dive deeper into the world of data observability. We’ll explore its components, tools, and pivotal role in enhancing software quality.
Discover our observability and reliability case studies that show how we’ve helped top brands achieve their business and development goals!
The Essence of Data Observability
At its core, it is about gaining a comprehensive view of the data flowing through our systems. It’s not just about monitoring; it’s about understanding the health, performance, and intricacies of our data pipelines.
This deep insight permits us to anticipate issues, make informed decisions, and maintain the integrity of our data ecosystem. The pillars of data observability (DO) allow for every aspect of our data’s lifecycle to be visible and actionable.
Expanding on this, DO delves into the granular details of data systems, enabling a level of transparency that was previously unattainable. By leveraging advanced tools, we can detect subtle changes in data quality, identify data lineage, and trace data issue root causes.
This proactive approach to managing data quality issues fosters a condition where our data assets remain a reliable foundation for decision-making.
Moreover, the benefits extend beyond mere problem-solving. It fosters a culture of accountability and continuous improvement among data teams. By making data health metrics visible and understandable, it encourages data engineers, data scientists, and all stakeholders to collaborate more effectively.
This synergy enhances the overall data quality and reliability, making DO an indispensable part of modern data management strategies. Now, let’s examine why this is so relevant in today’s data-driven environment.
Don’t miss this episode about observability on Quality Sense Podcast, with Federico Toledo and Lisa Crispin.
Why Data Observability Matters
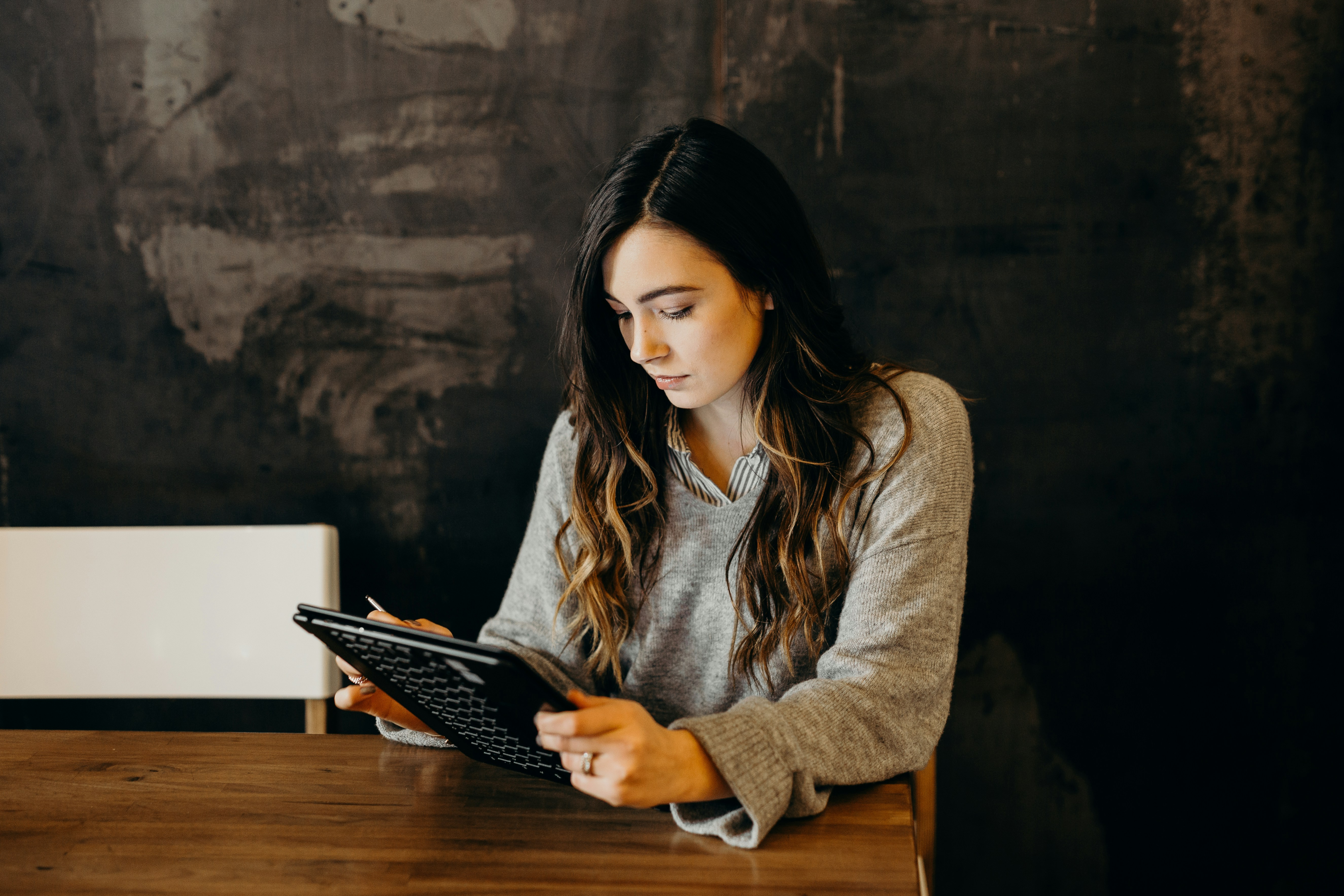
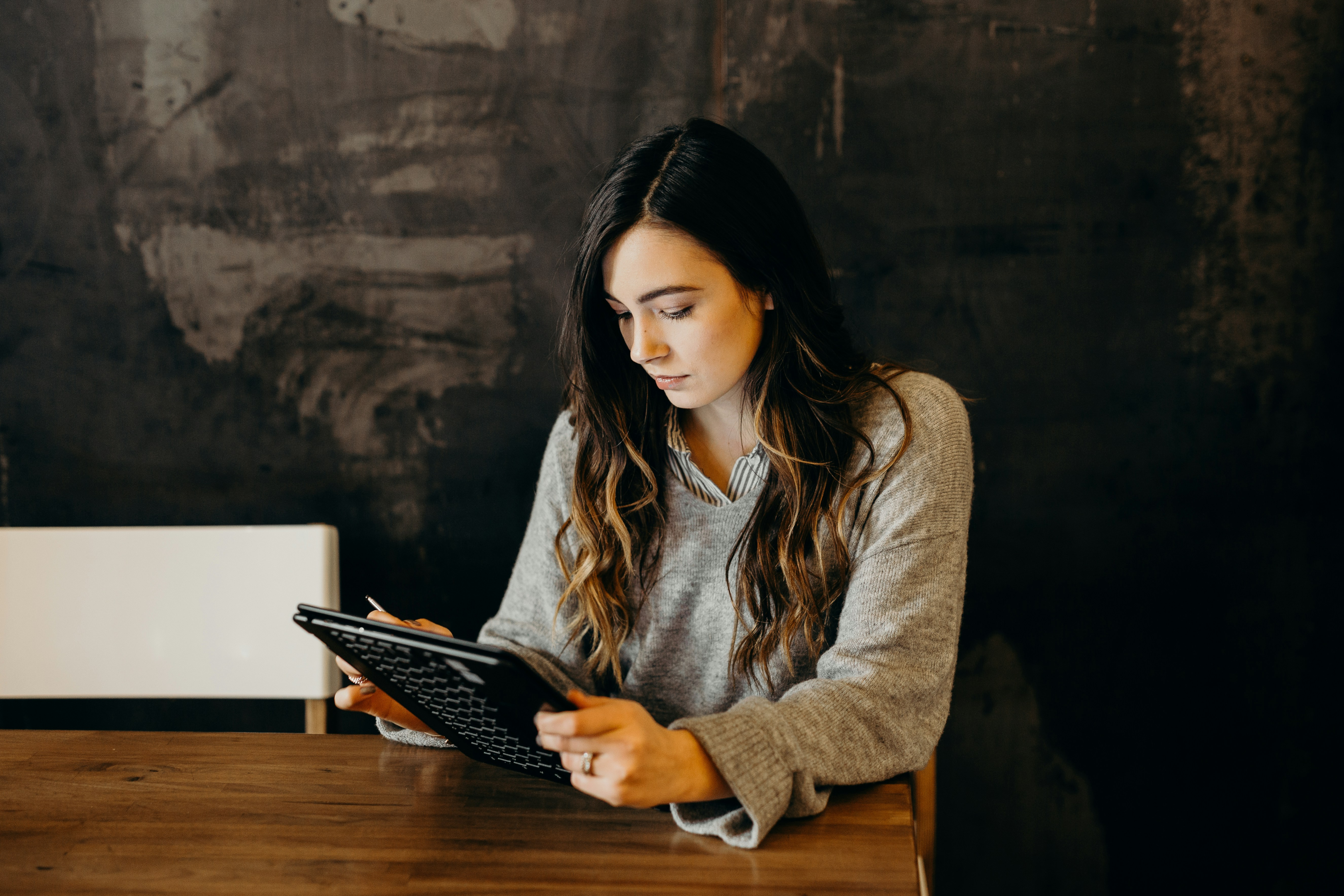
In an era where data is king, streamlining its quality and reliability is paramount. Here’s why data observability is crucial in today’s data-driven environment:
Empowers Data Teams:
Data observability empowers data teams to identify anomalies, and track data lineage. This enables the information we rely on for decision-making to be accurate and timely.
For example, consider a marketing team that relies on customer data to tailor campaigns. With data observability, they can quickly spot and correct any data discrepancies, allowing the campaigns to reach the right audience.
Goes Beyond Traditional Monitoring:
Data observability vs traditional monitoring reveals a broader scope, focusing not only on the operational aspects but also on the strategic value of data.
For instance, an e-commerce platform using traditional monitoring might detect a server outage. However, with DO, they can also track data flow issues that affect customer checkouts, preventing lost sales and improving user experience.
While traditional monitoring might alert us to system downtimes, DO provides a comprehensive view of data health, data downtime, and performance across the entire data lifecycle.
Boosts Data Reliability:
This holistic view is crucial for preventing data downtime and allowing for data reliability, which in turn, supports better business outcomes. Furthermore, data observability empowers data teams to manage the ever-increasing data volumes and complexity of modern data ecosystems.
For example, a financial services company can use it to monitor transaction data flows. They can quickly identifying and resolving issues that could lead to downtime or data inaccuracies, thereby maintaining customer trust and regulatory compliance.
Supports Advanced Data Practices:
As organizations adopt advanced machine learning models and data analytics, robust data observability solutions become even more critical. It promotes data to be of high quality, thereby elevating the accuracy and reliability of these advanced models.
Data observability is not just a tool or a process; it’s a critical component of a modern data strategy fostering reliability, accuracy, and timeliness of the information. Next, we’ll explore the tools of the trade that enable effective data observability.
Tools of the Trade
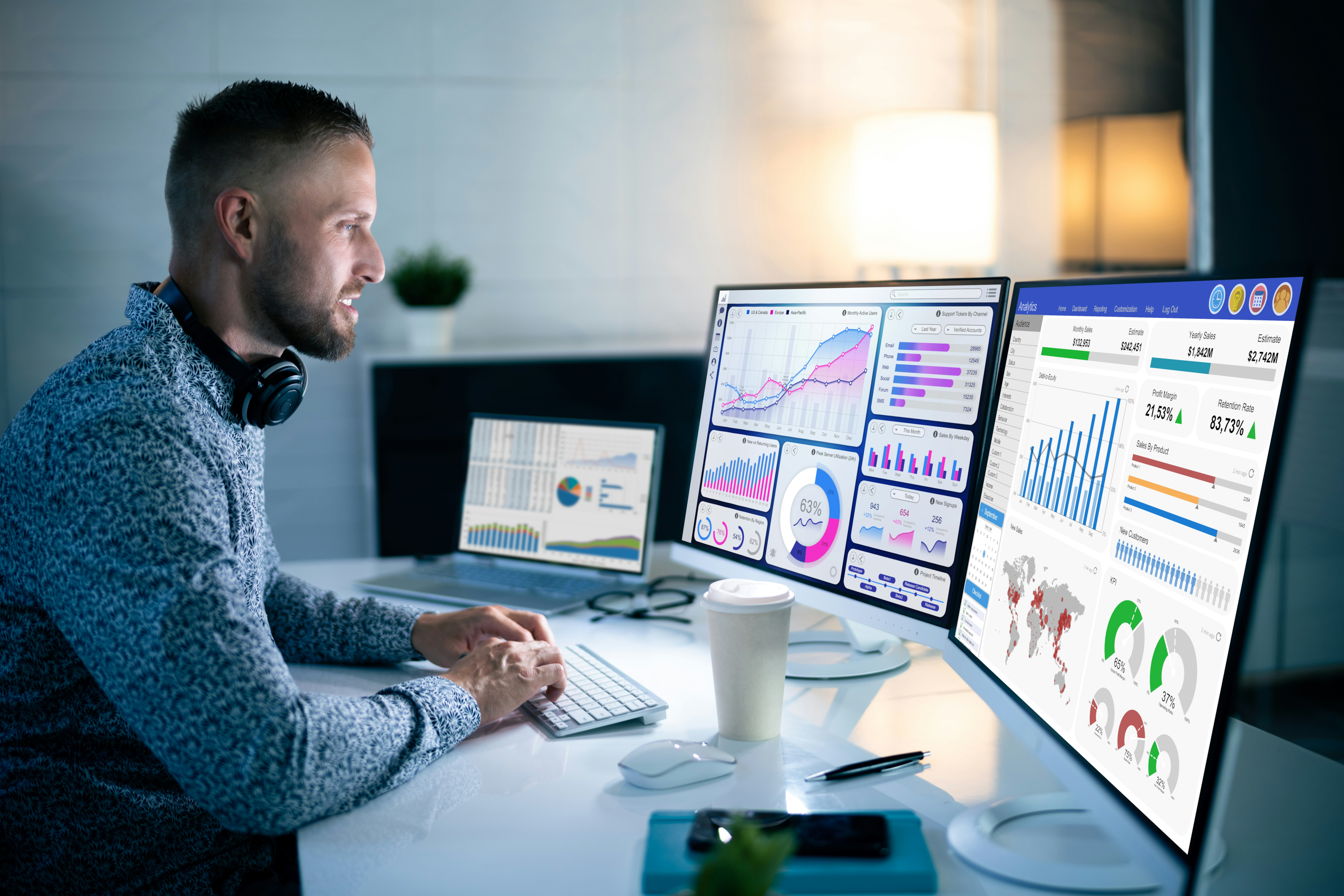
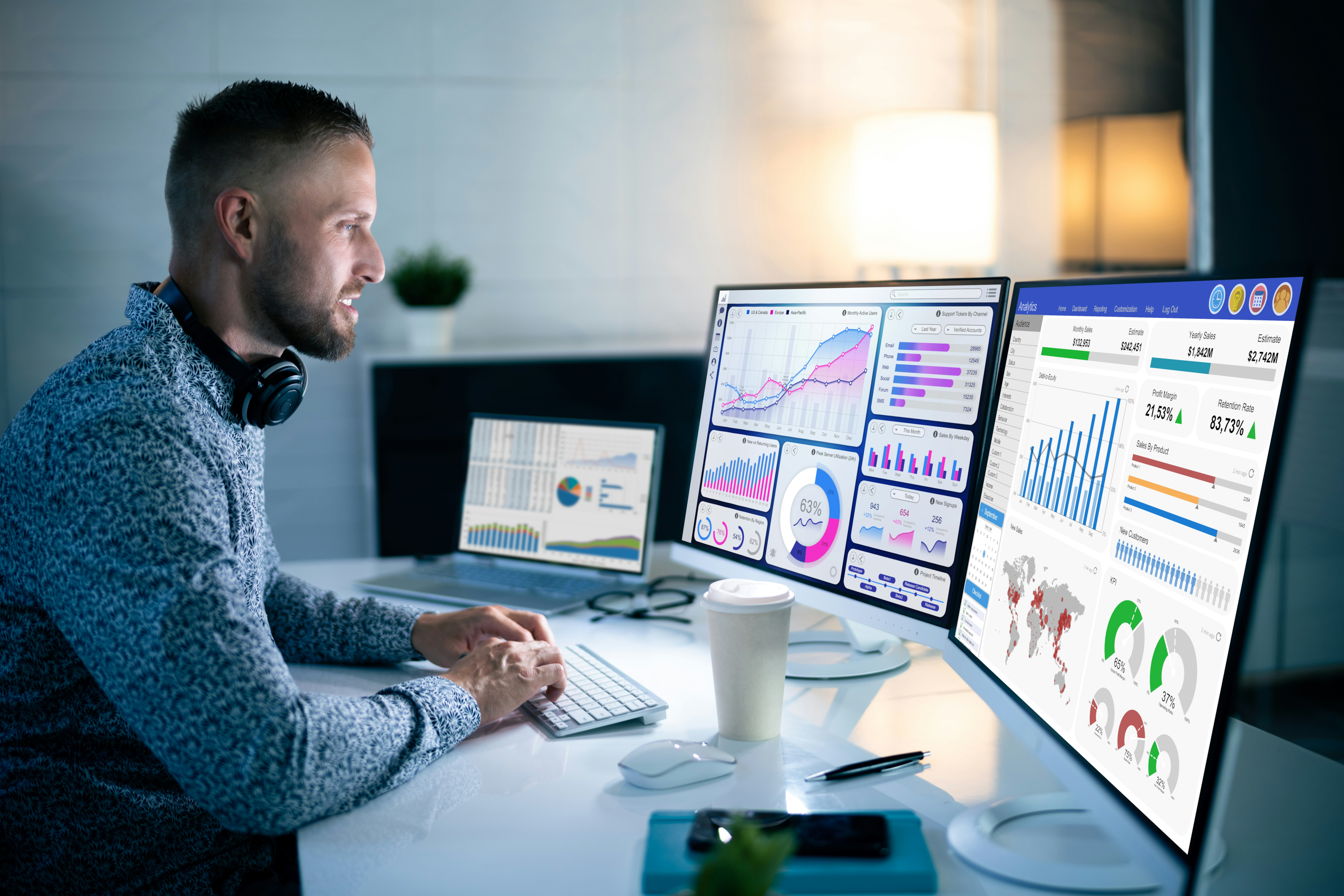
Navigating the landscape of data observability tools can be daunting. Yet, these tools are our allies, offering features like real-time monitoring, alerting, and detailed analytics. They enable us to dissect our data’s behavior, uncovering insights that drive optimization and improvement. The right data observability tool or platform can transform our data management practices.
Choosing the appropriate data observability tools is a critical decision for any organization. Not only should they provide comprehensive monitoring capabilities but also integrate seamlessly with existing data pipelines and data systems.
The best data observability platforms offer customizable dashboards, automated alerting systems, and in-depth analytics that cater to the unique needs of data engineering teams. This customization allows teams to focus on the metrics that matter most to their specific data environment and data processes.
In addition to technical capabilities, the selection of data observability tools should consider the ease of use and the learning curve for data professionals. Tools that offer intuitive interfaces and clear documentation can significantly reduce the time to value in their daily operations.
This user-friendly approach democratizes data observability, making it accessible to not just data engineers but also to data consumers across the organization. Let’s now focus on how it fosters data quality.
Fostering Data Quality
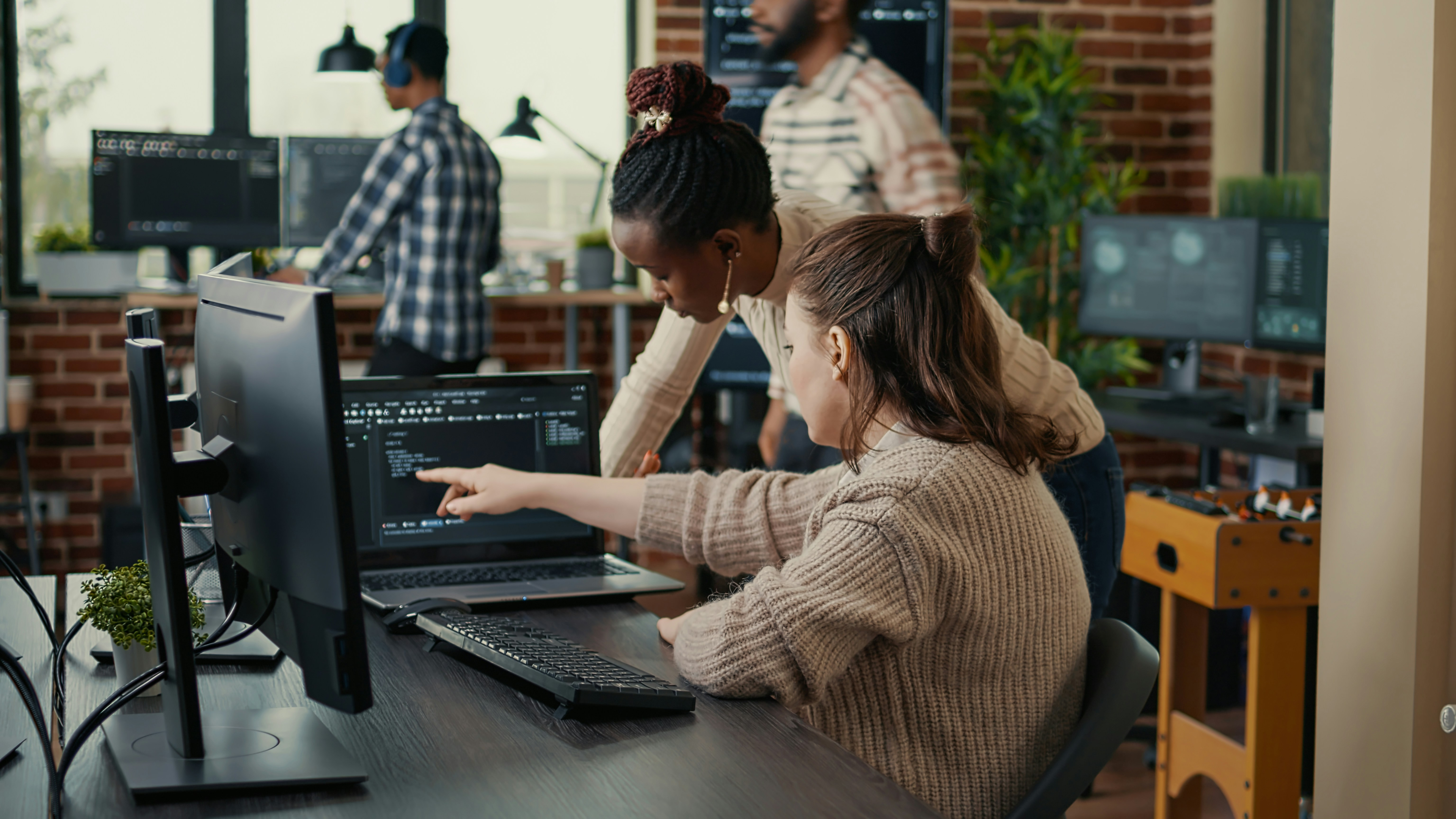
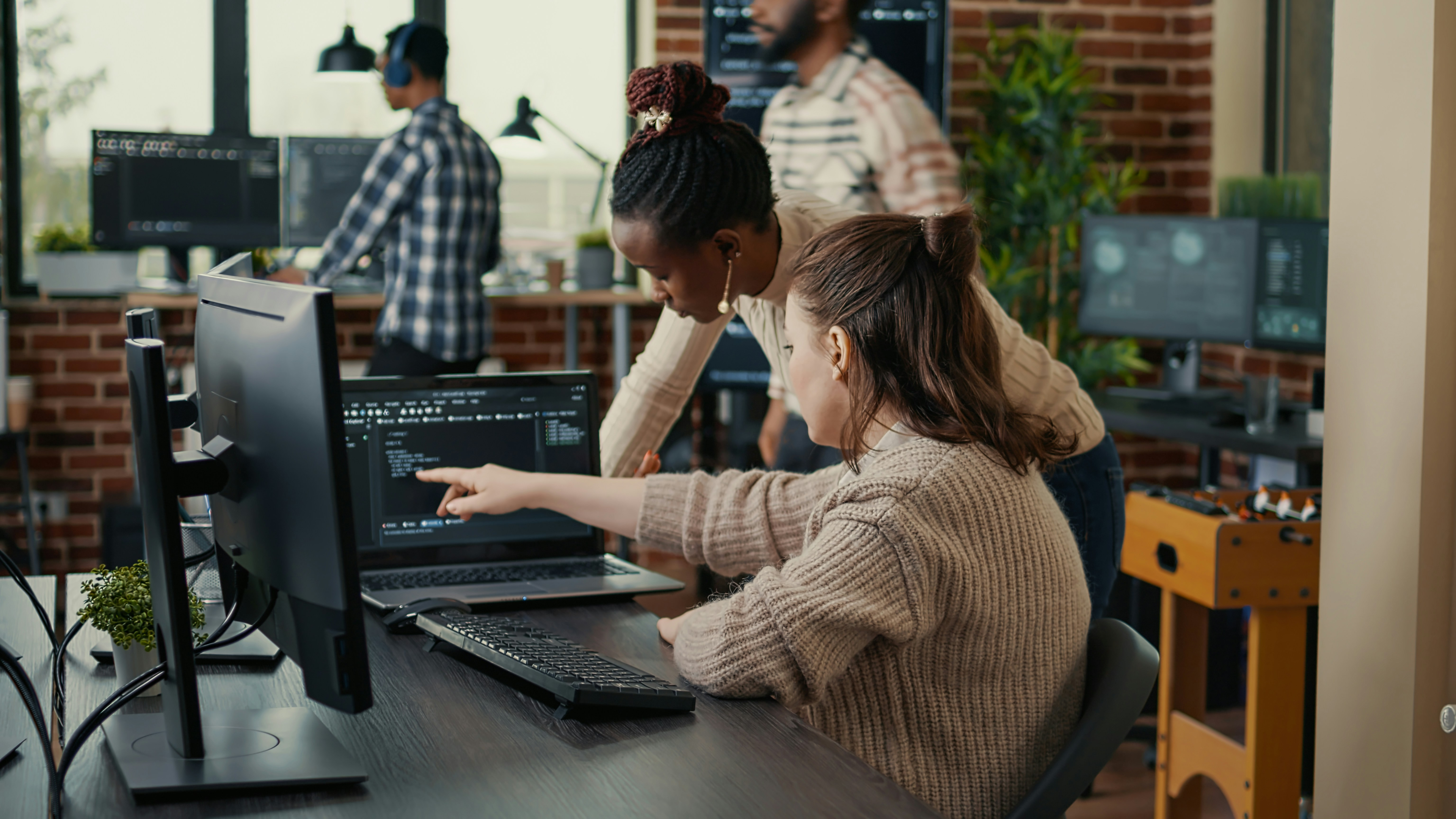
Data quality is not a destination; it’s a continuous journey. Through DO, we can implement checks and balances that enables our data to be accurate, consistent, and reliable.
This relentless pursuit of quality is what sets us apart in a data-driven world. Improving data quality is a critical outcome of effective data observability practices.
- Identifying and Addressing Data Quality Issues: Tools dedicated to DO play a crucial role in detecting and diagnosing data quality problems. By continuously monitoring data quality metrics, these tools can alert data teams to issues such as missing data, duplicate records, or inconsistencies that may impact data quality.
- Enhancing Data Reliability: Data reliability is closely tied to data quality. High-quality data must be reliable, meaning it is available and accurate when needed. Data observability solutions promote the monitoring of data pipelines for performance bottlenecks and errors, reducing data downtime and enhancing overall data reliability.
- Supporting Data Governance Initiatives: Observability solutions extend their utility to the realm of data governance by shedding light on the origins and pathways of data. This visibility and transparency is indispensable for meeting regulatory compliance standards and safeguarding the integrity of an organization’s data repositories.
By focusing on these key areas, organizations can maintain high-quality data, the foundation for each data-driven decision. A key component of this process is the data pipeline, the backbone of our operations.
The Backbone: Data Pipeline
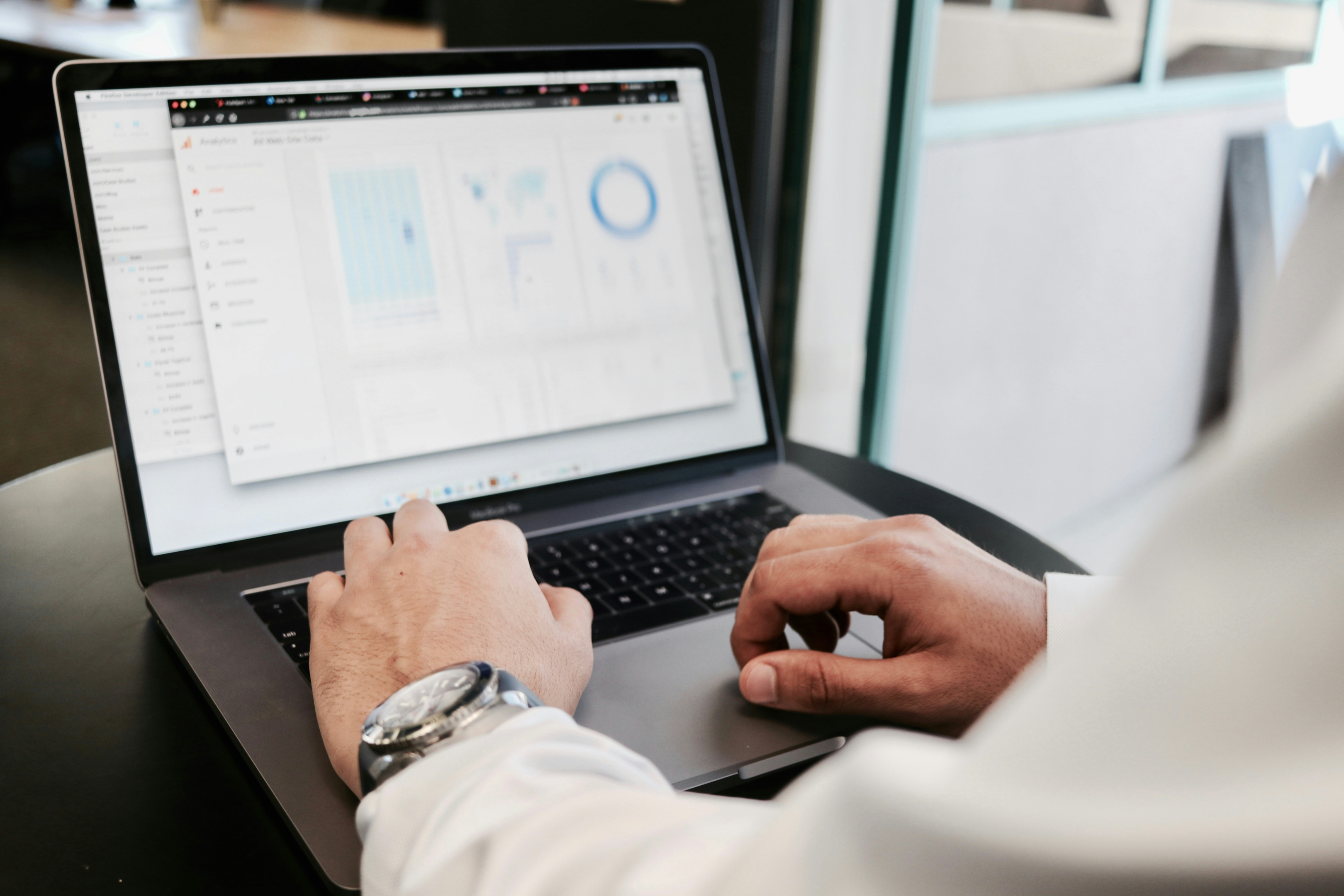
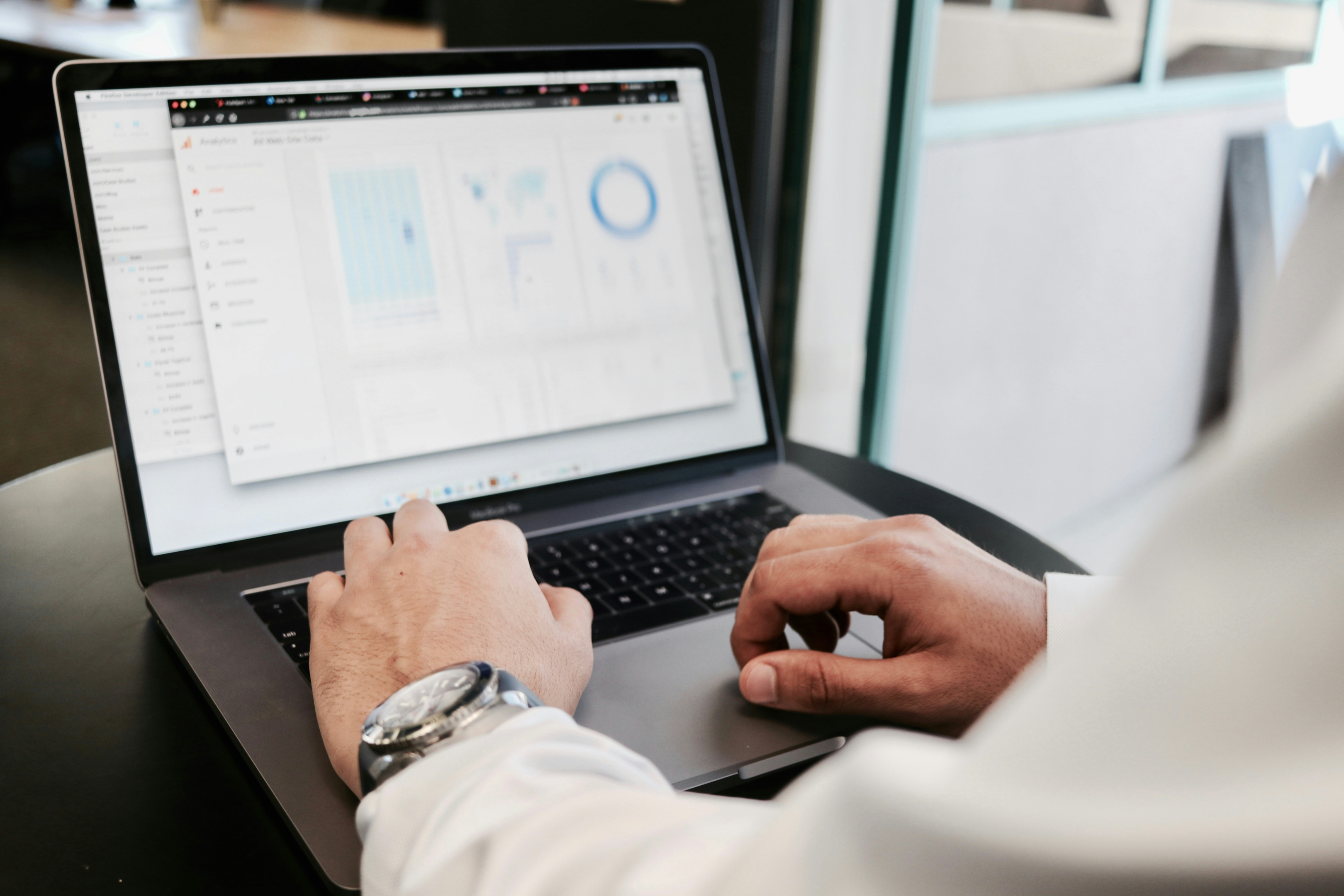
Our data pipeline is the backbone of our operations, a critical infrastructure that demands our attention. Data observability shines a light on every stage of the pipeline, from ingestion to processing and storage, ensuring that each step functions seamlessly and efficiently.
Data pipeline monitoring is an essential component of data observability, enabling us to maintain high-quality data throughout the entire data lifecycle.
Real-World Example:
Imagine a scenario where an e-commerce company experiences sudden drops in sales. By leveraging data observability tools, the data team quickly identifies a bottleneck in the data pipeline that processes customer transactions.
The issue is traced back to a recent update in the data processing algorithm, which inadvertently filtered out valid transactions. With this insight, the team promptly rolls back the change, restoring the data flow and preventing further sales loss.
It’s also important to distinguish between DO and traditional monitoring to understand the full scope of its benefits.
Data Observability vs. Traditional Monitoring
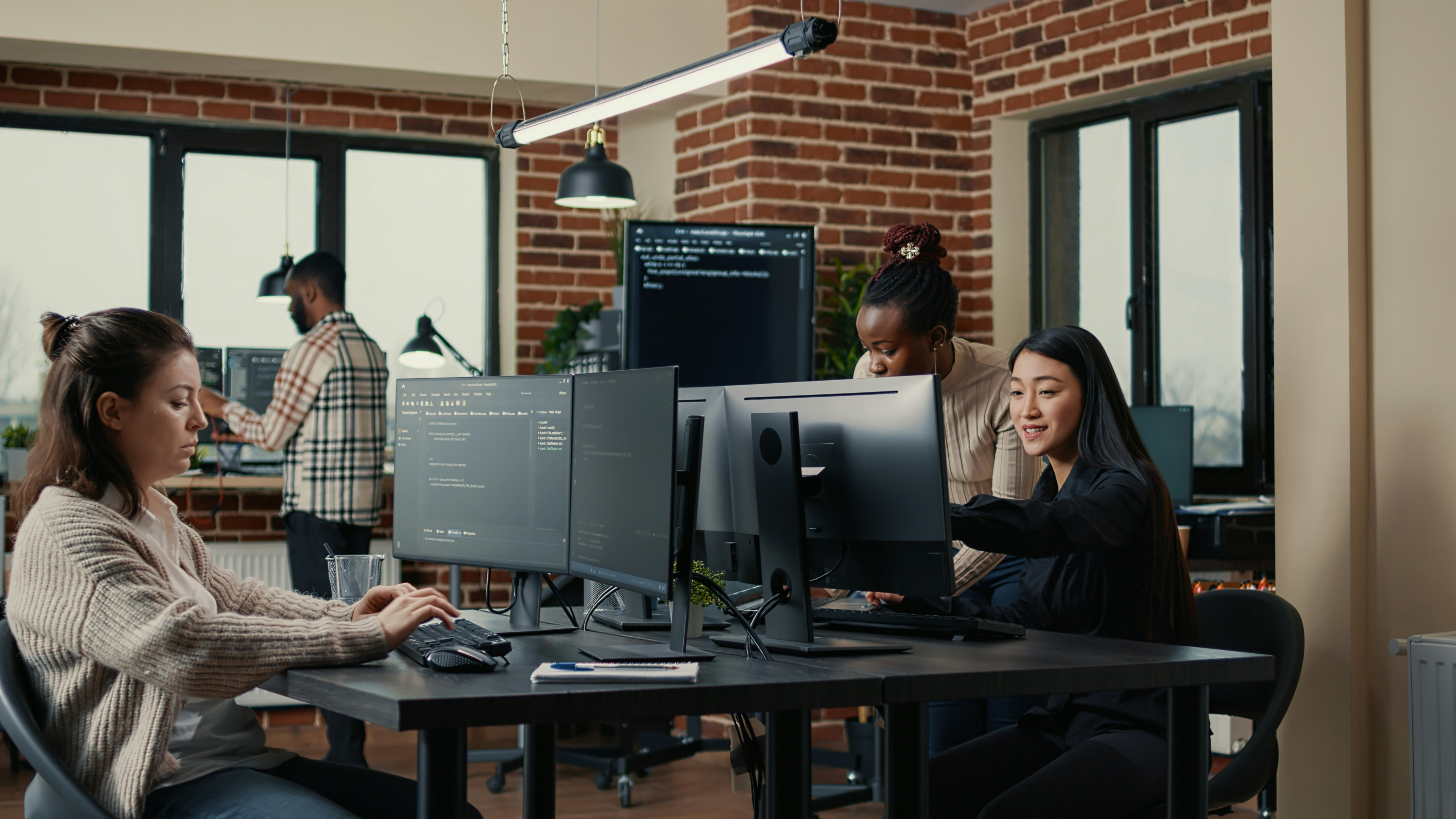
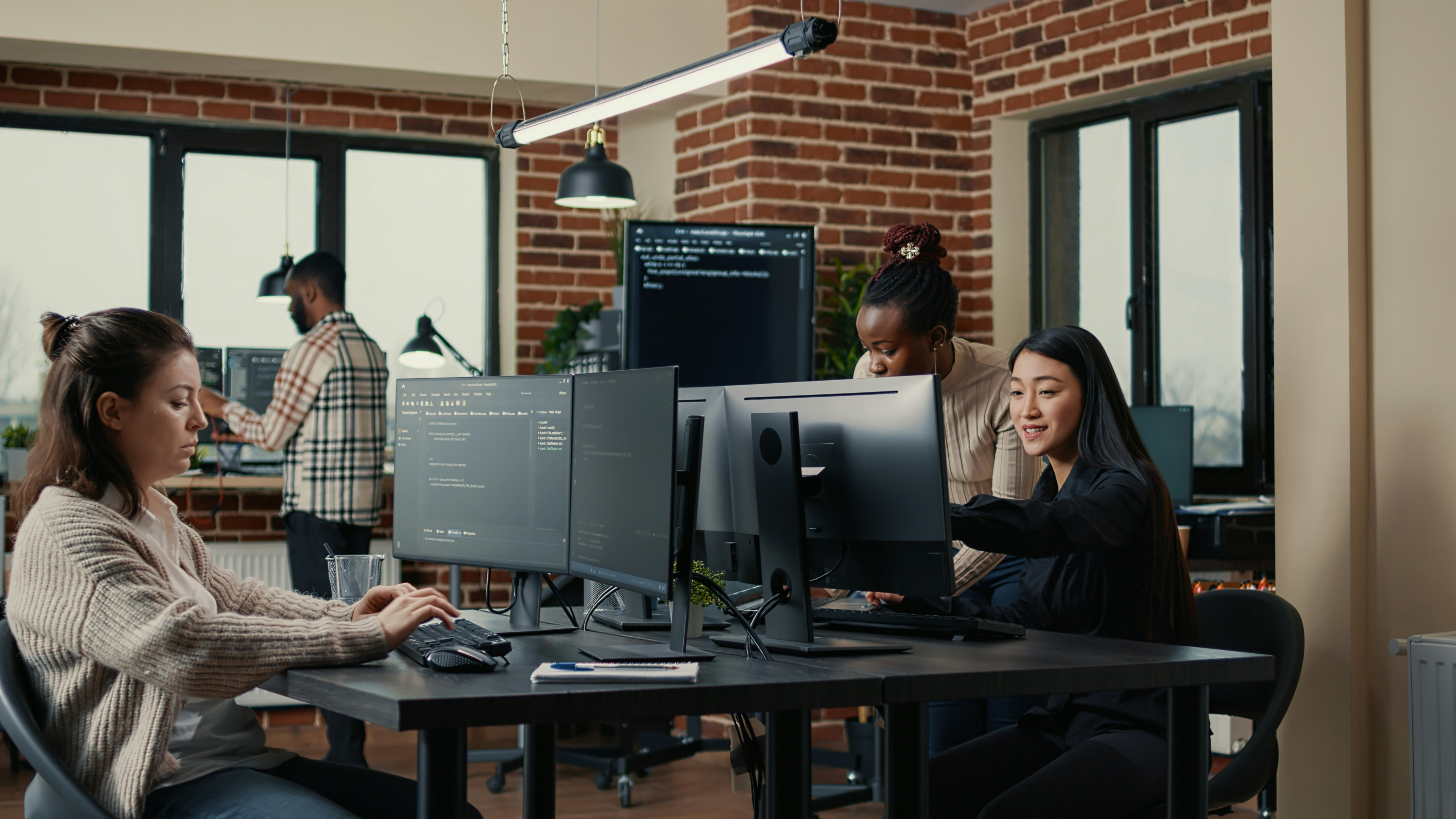
It’s important to distinguish between data observability and traditional monitoring. While both are essential, data observability offers a more comprehensive view of our data ecosystem, enabling root cause analysis and proactive management of data quality issues.
This holistic approach is what sets data observability apart, making it a key strategy in modern data management.
Proactive vs. Reactive
Traditional monitoring often takes a reactive approach, alerting teams after an issue has occurred. Data observability, on the other hand, aims to be proactive, identifying potential problems before they impact the system.
Depth of Insight
While monitoring might tell you that a system is down, DO seeks to explain why it happened by providing deeper insights into data health, data flows, and system interactions.
Scope of Visibility
Traditional monitoring focuses on operational metrics like uptime and performance, whereas data observability encompasses a broader range of data metrics, including data quality, data lineage, and data reliability. This broader scope significantly empowers data teams.
Empowering Data Teams
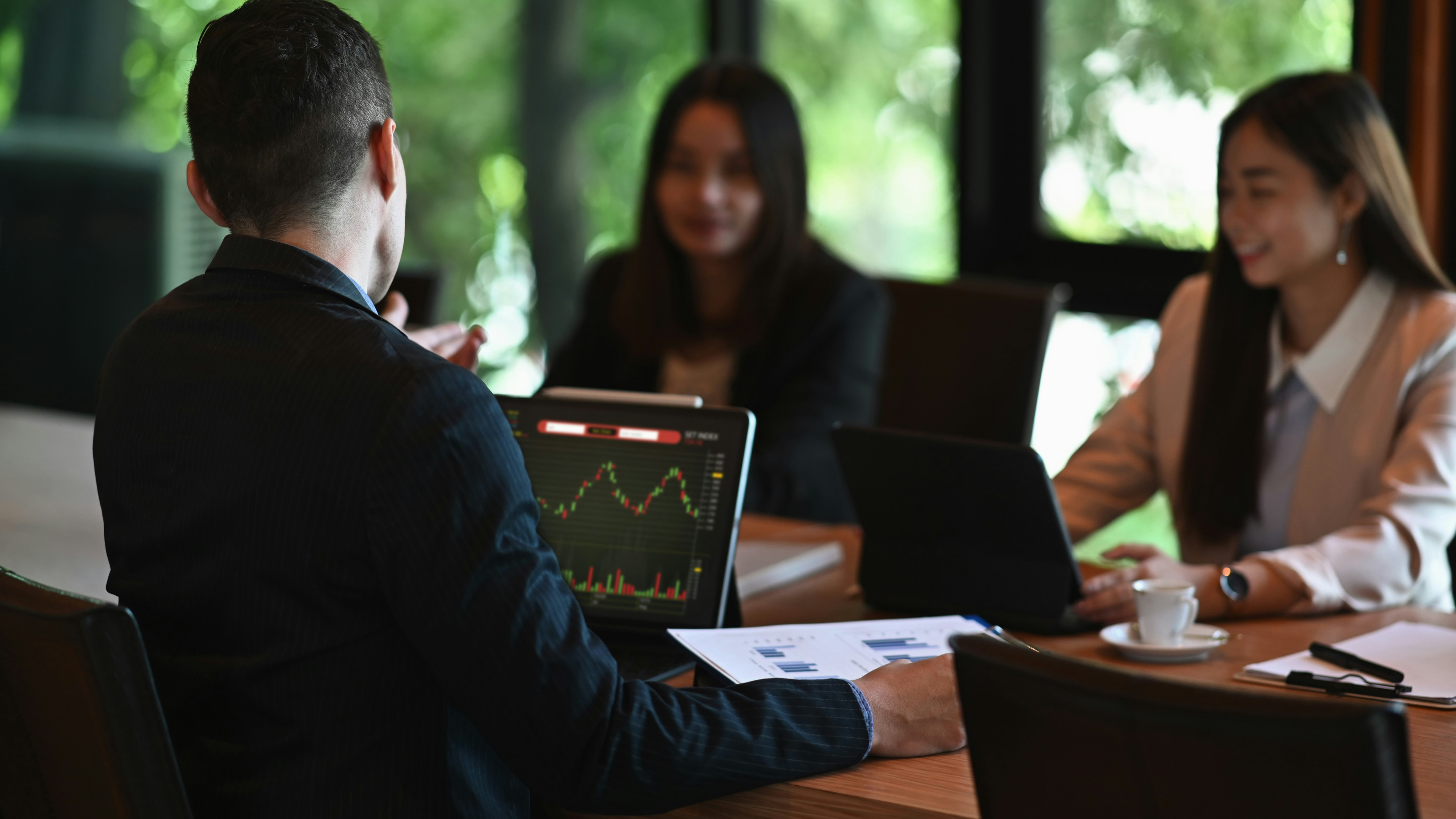
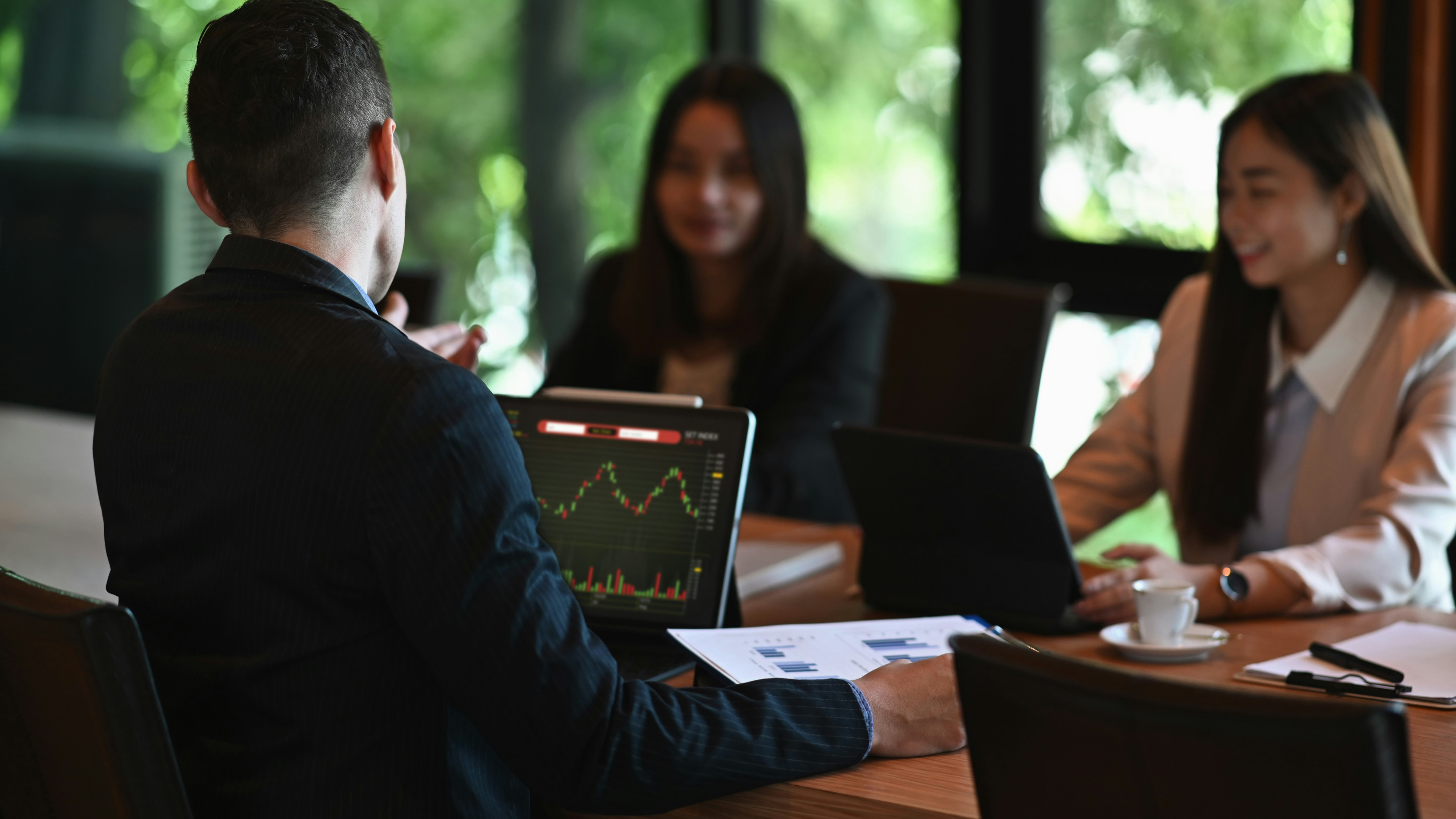
Data observability empowers data teams by providing them with the tools and insights needed to manage and improve the data environment effectively.
From data engineers to data scientists, every member of the team benefits from enhanced visibility into the data lifecycle, enabling better decision-making and more efficient data operations.
- Facilitating Collaboration: Data observability fosters a collaborative environment where data engineers, data scientists, and business analysts can work together more effectively. Shared visibility into data health and performance metrics encourages cross-functional teams to collaborate on solving data issues and optimizing data processes.
- Driving Innovation: With a comprehensive understanding of the data ecosystem, data teams are better positioned to innovate. Data observability provides the insights needed to experiment with new data models, machine learning algorithms, and analytics techniques, driving forward the organization’s data strategy.
- Business results: Being able to have a wide data visibility over different stages, harness teams to correlate business results with system’s observable data and insights.
Some Questions for Reflecting
- Can you recall an instance where the implementation of data observability might have averted a significant data-related challenge within your organization?
- In what ways do you anticipate data observability will adapt as cloud technologies and distributed data systems become more prevalent?
We invite you to share your insights and experiences in the comments section below! Looking ahead, let’s explore the future of data observability and its evolving role.
Looking Ahead
With the advent of more complex data systems and the increasing reliance on data-driven decision-making, the need for comprehensive data observability strategies will be increasingly critical.
- Integration with AI and Machine Learning: Data observability platforms will increasingly leverage AI and machine learning models to predict potential issues and automate root cause analysis, further enhancing the ability of data teams to maintain high-quality data.
- Expansion Beyond Technical Teams: Data observability will become a shared responsibility across the organization, with non-technical stakeholders gaining access to observability insights. This democratization of data will empower entire organizations to make informed decisions based on data health and performance metrics.
- Enhanced Data Governance and Compliance: As data privacy and compliance regulations continue to evolve, data observability will play a crucial role in ensuring that organizations meet these requirements. By providing transparency into data sources, movements, and transformations, data observability supports robust data governance frameworks.
Final Thought: Embracing Data Observability
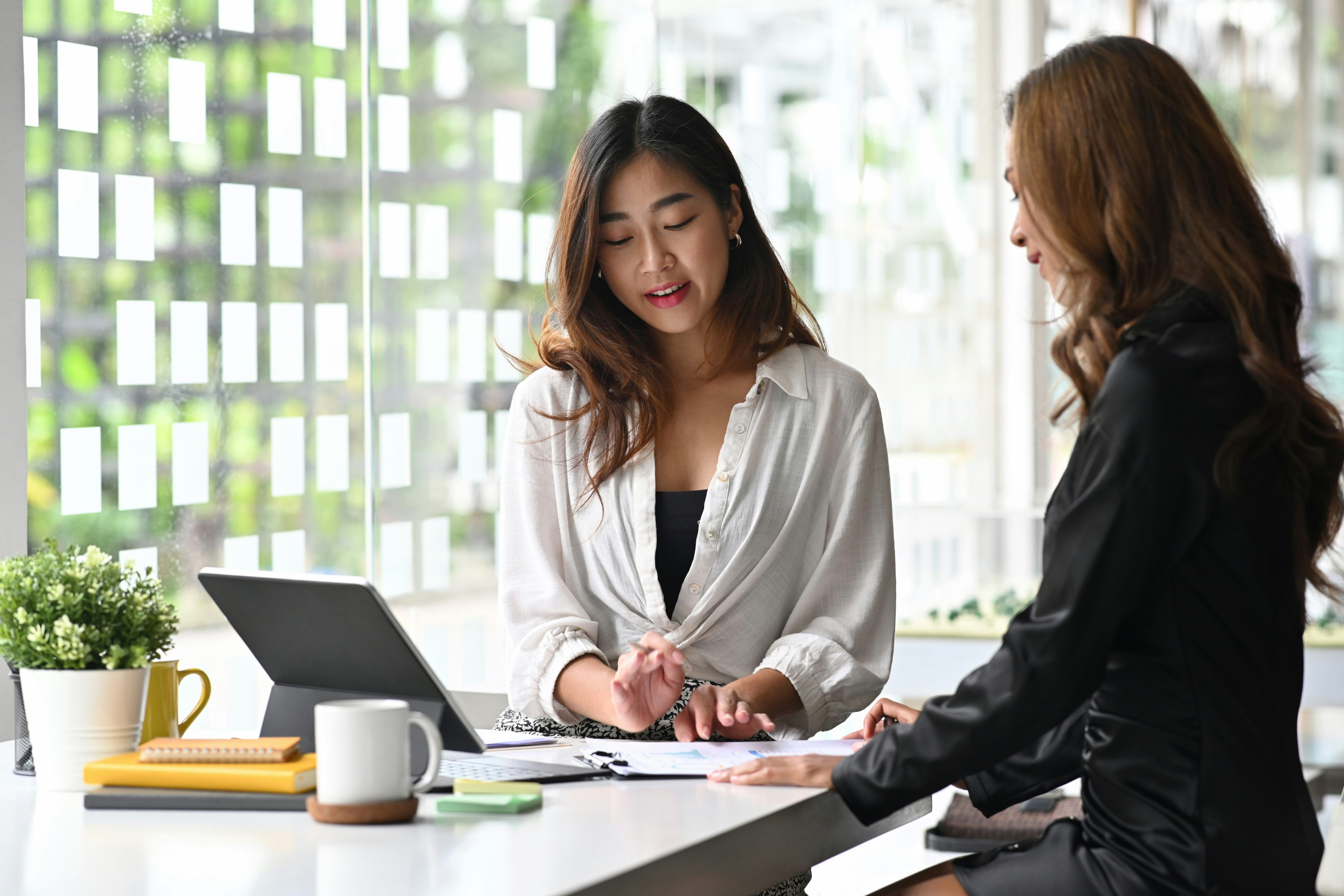
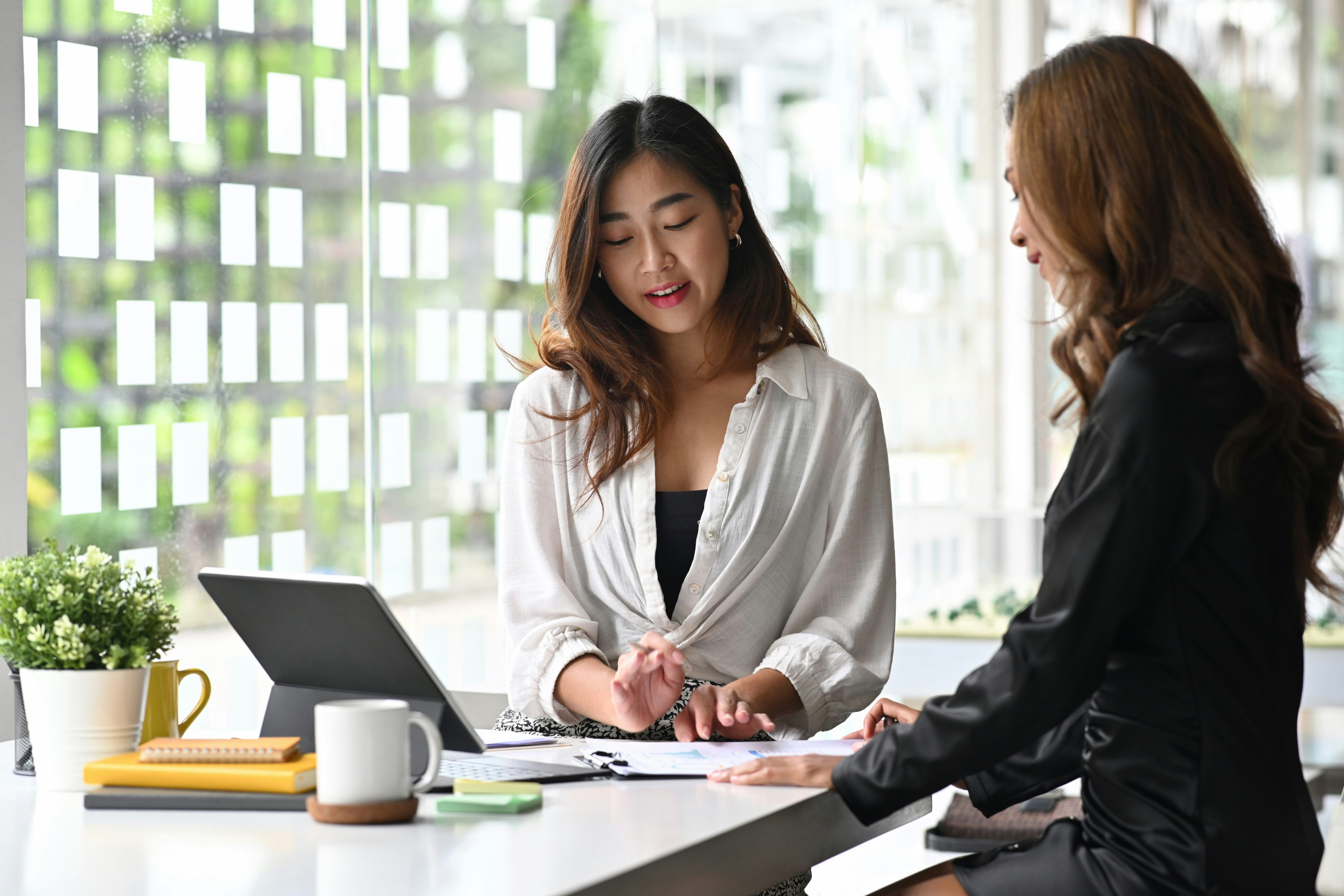
In conclusion, the journey toward effective data observability is both a challenge and an opportunity. It requires a shift in mindset from reactive monitoring to proactive management of data ecosystems.
By embracing it, organizations can unlock the full potential of their data, promoting its quality, reliability, and value in driving business decisions.
This is not just a set of tools or practices; it’s a foundational element of a modern data strategy. As we continue to navigate the complexities of today’s data landscapes, let us commit to fostering a culture of transparency, collaboration, and continuous improvement.
Together, we can harness the power of DO to build more resilient, data-driven organizations.
We invite you to read this article: Why Abstracta’s Professionals Can Elevate Your Software Quality.
How We Can Help You
With over 15 years of experience in the field, Abstracta is one of the most trusted companies in quality engineering.
Observability is crucial to achieving quality software development. Having an alliance with Datadog, we will have enhanced our observability capacity through its infrastructure monitoring and analysis tools, with greater efficiency and quality of work. Discover more benefits of our partnership in this article!
Partnering with us means having access to the best tools ever and counting on our leaders’ expertise. Thanks to our partnerships, we have the opportunity to provide our customers with more complete solutions and first-class products.
Looking for expert assistance? We are here to provide you with top-notch services and solutions tailored to your unique needs. Contact us to discuss how we can help you grow your business
FAQs
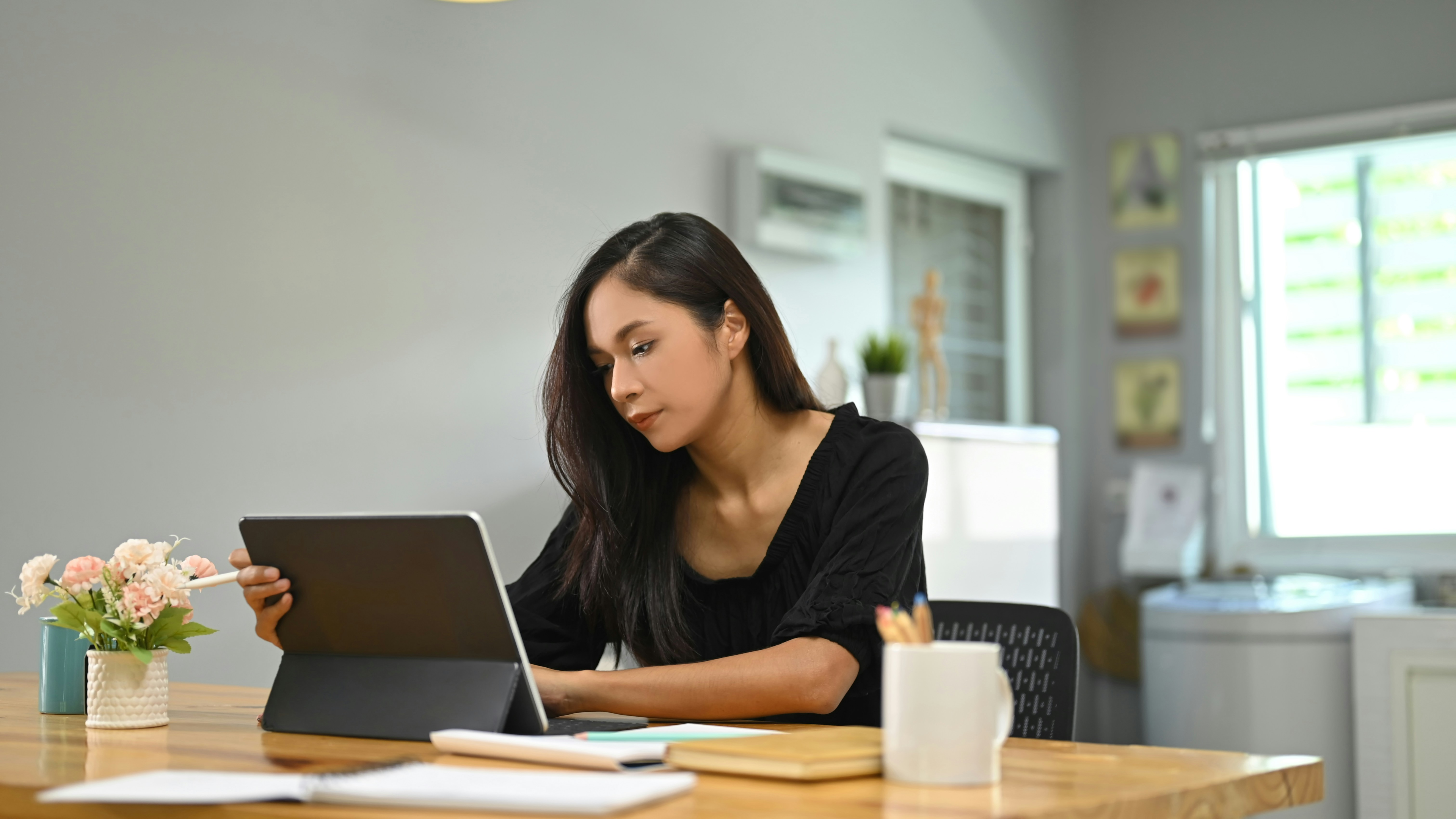
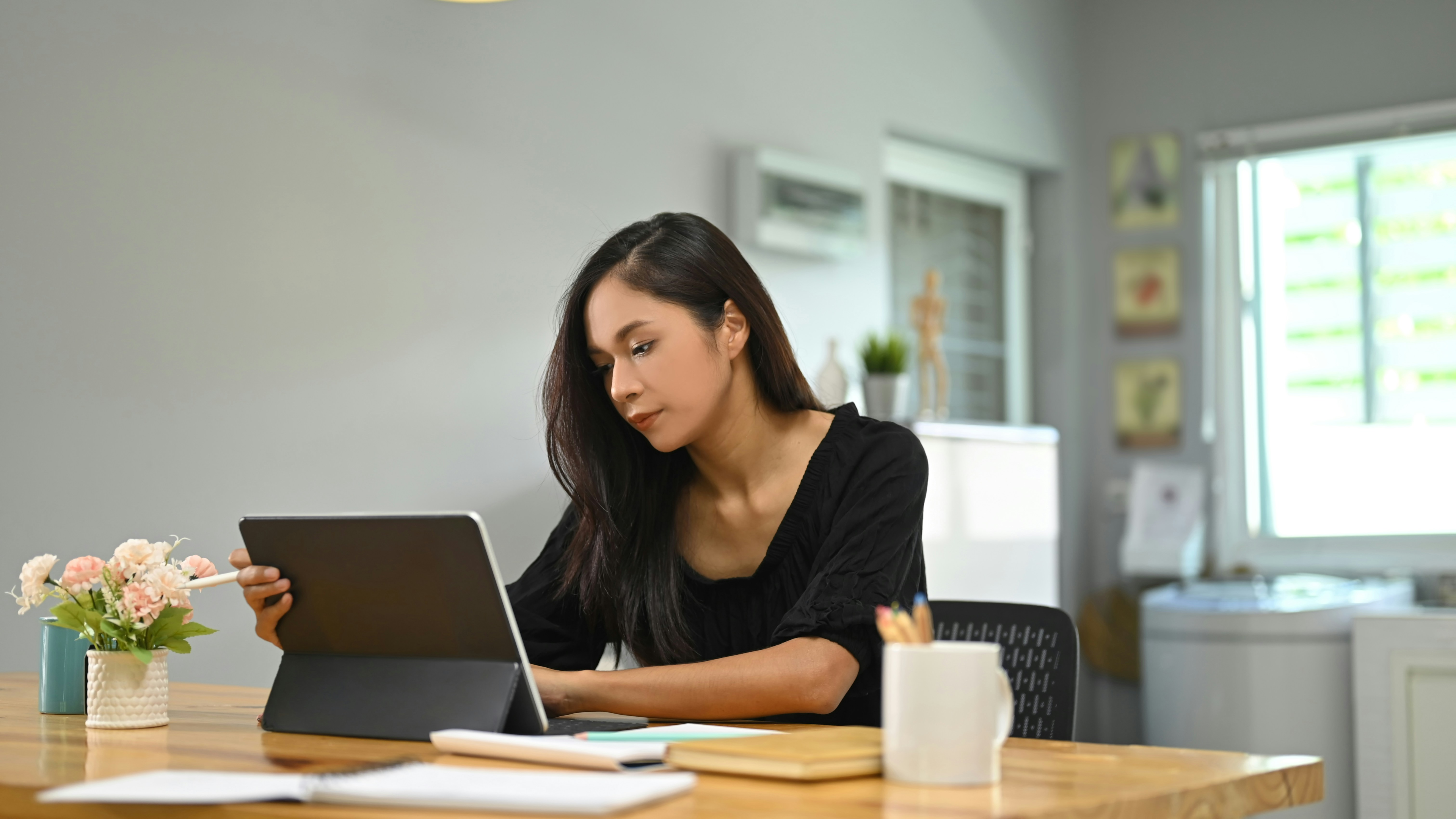
What Do You Mean by Data Observability?
It refers to the ability to fully understand the health, quality, and performance of data within an organization’s systems. It involves monitoring, tracking, and diagnosing data through its lifecycle to ensure it remains accurate, reliable, and accessible for decision-making processes.
Incorporating data monitoring techniques, DO provides a comprehensive view of the data ecosystem, enabling proactive identification and resolution of issues.
What Are The 4 Pillars of Data Observability?
1. Freshness: How up-to-date your data is.
2. Distribution: The patterns and anomalies within your data values, enabling them to meet expected ranges and behaviors.
3. Volume: Monitoring changes in data volumes to catch issues like data loss or unexpected influxes.
4. Schema: Tracking changes in a data structure or schema to prevent integration and analysis problems.
All these pillars together support a robust framework for understanding and improving data health and reliability.
What is The Difference Between Data Discovery and Observability?
Data discovery and observability serve different purposes in data management. Data discovery is the process of identifying and understanding data assets within an organization, often focusing on locating and categorizing data.
On the other hand, DO goes beyond discovery to continuously monitor and analyze data quality, performance, and reliability throughout its lifecycle. While discovery lays the foundation for knowing what data you have, observability ensures that this data is healthy and usable.
What Is The Difference Between Data Quality and Data Observability?
Data quality refers to the condition or fitness of data to serve its intended purpose, focusing on aspects like accuracy, completeness, and consistency.
Data observability, meanwhile, is a broader concept that encompasses the monitoring and management of data quality, among other attributes like performance and reliability.
While ensuring data quality is a goal, data observability provides the means to achieve and maintain this goal by offering insights into the health of data across its entire lifecycle.


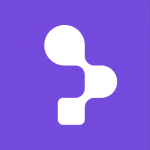
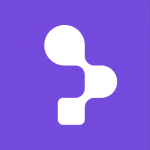
Abstracta Team
Related Posts
Quality Sense Podcast: Lisa Crispin – Software Observability
The who, what, why, and how of observability for teams building software In this Quality Sense episode, host, Federico Toledo, sits down for a virtual chat with Lisa Crispin. She’s recently assumed the role of Quality Owner at the low-code development platform, OutSystems, which is…
Quality Sense Podcast: Alon Girmonsky – Testing Microservices with UP9
Challenges of testing microservices and why we need observability In this episode of Quality Sense, host, Federico Toledo intervews Alon Girmonsky, an accomplished, multi-time entrepreneur and technology leader. He’s a passionate engineer who loves building developer tools and companies. Today, he’s the CEO and co-founder…
Leave a Reply Cancel reply
Search
Contents